Home
Learn From The Commerce Execution Leaders.
Latest Articles
Striking a Balance: Uniting Category Management and Pricing Team for Retail Success
In retail operations, the interaction between category management and price optimization teams is key to staying competitive. This dynamic, often.
- by Mollie Panzner
- April 25, 2024
Real-Time Insights Drive Success in New Ice-Cream Product Launch
A national ice cream manufacturer sought to make an impact in the market with the launch of four new SKUs..
- by Matt Ellsworth
- April 24, 2024
Popular Topics
Browse Our Guides

Brand Management: How to Create a MAP Policy, Implement MAP Monitoring, and Protect Your Brand

Editor's Choice
News From Wiser
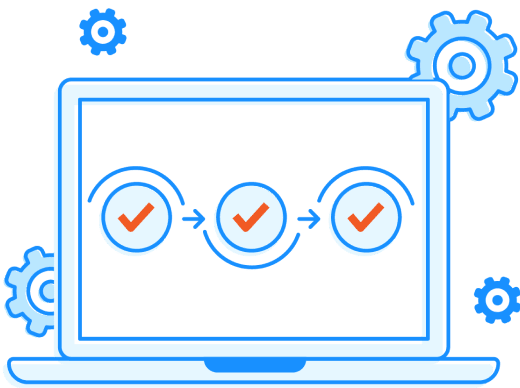